AI Literacy is the ability to understand, use, and think critically about artificial intelligence systems and their impact on our world.
Just as we’ve become comfortable with terms like ‘prompt’, ‘ChatGPT’, and ‘hallucination’, the language of AI continues to evolve. Understanding these terms isn’t just for tech experts anymore—it’s becoming an essential skill for anyone wanting to participate effectively in our AI-powered world.
I have selected 12 essential terms that I think everyone should start getting comfortable with. For each term, I’ve included both a clear definition and a relatable analogy to help you grasp the concept quickly. Think of this as your friendly guide to speaking “AI”—no technical background required.
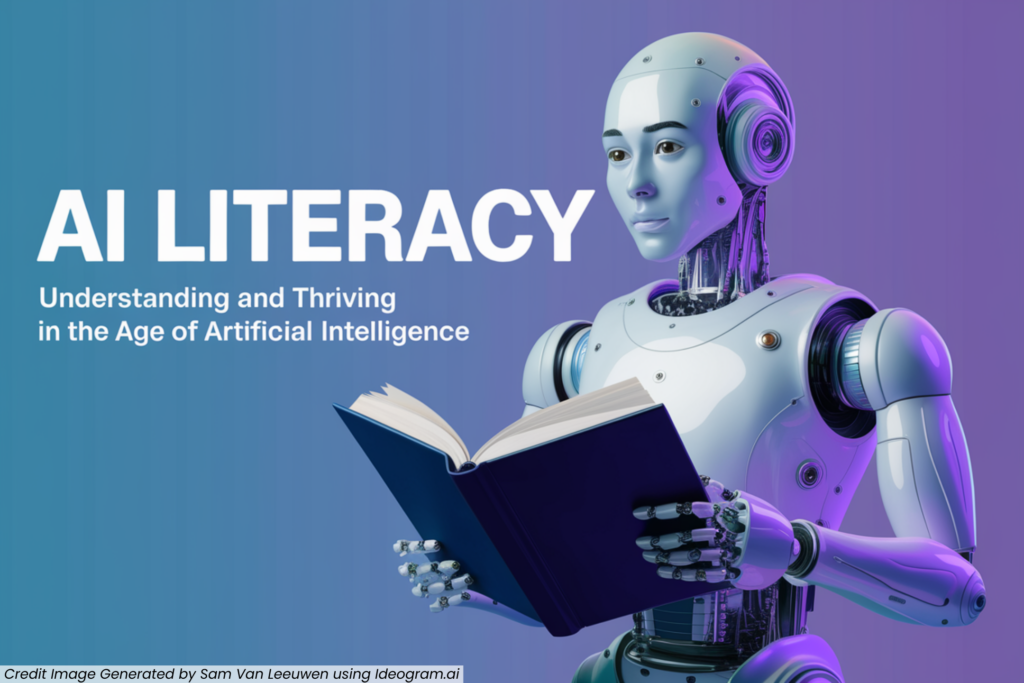
Here are the '12 AI terms everyone should know'
If you prefer audio content, here’s a short podcast on the AI literacy terms created by our team using NotebookLM:
AI vs Machine Learning
Artificial Intelligence is the broader concept of machines being able to perform tasks intelligently, while Machine Learning is a specific approach where systems learn from data rather than being explicitly programmed.
Think of Artificial Intelligence as the entire concept of machines being smart and making decisions—like the whole idea of building a robot butler. Machine Learning is one way to achieve this: instead of programming every action, we let the machine learn from examples, like teaching that butler by showing it thousands of videos of how to do tasks rather than writing step-by-step instructions.
Machine Learning vs Deep Learning
Deep Learning is a specialised type of Machine Learning that uses layered neural networks to automatically discover important features in data without human guidance.
If Machine Learning is teaching our robot butler by showing examples, Deep Learning is teaching it to think more like a human brain. While basic Machine Learning might need help identifying important features (like “doors have handles”), Deep Learning can figure out these patterns on its own through layers of processing—similar to how our brains build up understanding from simple details to complex concepts.
Training
Training is the process of feeding data into an AI system to help it learn patterns and relationships that it can later use to make decisions or predictions.
Training is like the AI’s “education phase,” where it learns by studying vast amounts of data, similar to a student preparing for exams. Some AI models study broadly across internet data like Wikipedia (generalist), others focus on specific industries like tax law (vertical), while some are trained exclusively on a company’s private data (company specific).
Inference
Inference is the process where an AI model applies what it learned during training to make predictions or decisions about new, unseen data.
Inference is how AI applies its training in different situations—like a graduate using their skills in various jobs. Whether it’s a voice assistant responding instantly (real-time), a streaming platform updating recommendations in bulk (batch), facial recognition unlocking your phone (edge), or a chatbot tackling new questions it hasn’t seen before (zero-shot), inference shows how AI adapts its knowledge to match our different needs.
Reasoning
Reasoning is an AI’s ability to process information logically, make connections between concepts, and arrive at meaningful conclusions.
Reasoning in AI is the ability to understand information and make logical decisions, going beyond simple pattern matching. Think of early AI models like students who could only memorise answers—now, with reasoning capabilities, AI can “think through” information like a student who truly understands the material, making it more flexible for complex real-world tasks.
Large Language Models (LLMs)
Large Language Models are AI systems trained on massive amounts of text data that can understand, generate, and manipulate human language in sophisticated ways.
Think of Large Language Models as comprehensive digital libraries with librarians who understand every book they’ve ever read. These massive AI systems, trained on vast amounts of internet text, can understand and generate human-like text, engage in conversations, write code, and even reason about complex topics—like having an expert in countless fields at your fingertips.
Small Language Model (SLMs)
Small Language Models are compact AI systems designed to perform specific language tasks efficiently with fewer computational resources.
Think of Small Language Models like pocket guides—compact, focused, and quick to access. Unlike their larger counterparts (the encyclopedias), SLMs are designed to be lighter and more efficient, perfect for specific tasks or devices with limited processing power.
Multimodal Models
Multimodal Models are AI systems that can process and understand multiple types of input and output, such as text, images, audio, and video simultaneously.
Multimodal Models are like having a renaissance expert who can seamlessly work across different forms of communication. They can understand and create connections between various types of input—text, images, audio, and video—similar to how humans naturally process information through multiple senses.
Retrieval Augmented Generation (RAG)
RAG is a technique that allows AI models to supplement their knowledge by accessing and incorporating information from external sources in real-time.
RAG is a method where AI connects to external sources for accurate, up-to-date information without needing retraining. Like ‘Phone a Friend’ on Who Wants to Be a Millionaire, it allows AI to instantly check trusted sources when it needs precise, verified information for its responses.
Prompt Engineering
Prompt Engineering is the practice of crafting and optimising input instructions to get the best possible outputs from AI models.
Think of prompt engineering as learning the art of asking the right questions. Just as a skilled interviewer knows how to draw out the best responses from people, prompt engineering is about crafting instructions that help AI models understand exactly what you want—turning “make me a website” into a detailed set of requirements that guide the AI to create exactly what you need.
Fine-tuning
Fine-tuning is the process of taking a pre-trained AI model and further training it on specific data to optimise it for particular tasks or domains.
Fine-tuning is like sending an experienced professional back to school for a specialisation. You take a model that’s already well-trained (like GPT or BERT) and give it additional training on specific data or tasks—similar to how a general practice doctor might specialise in pediatrics through focused training.
Constitutional AI
Constitutional AI is an approach to developing AI systems with built-in constraints and values that guide their behavior and decision-making.
Constitutional AI is like installing guardrails and values in an AI system from the start. Instead of trying to correct problematic behavior after the fact, it’s about training AI models with built-in principles and boundaries—similar to how we teach children values and ethics alongside their ABCs.
Is your team ready to move beyond the basics and develop practical AI literacy?
From understanding key concepts to applying AI tools effectively, our expert-led training programs help teams build the confidence and capabilities they need.
Speak with an AI expert and discover how we can tailor training for your businesses needs.