Imagine a world where AI doesn’t just predict what might happen, but actively makes decisions to achieve specific goals—from self-driving cars navigating busy intersections to investment algorithms adapting to market volatility in real-time. This isn’t science fiction; it’s the reality of reinforcement learning, and it’s transforming how businesses operate today.
As we explored in our recent article “2025: The Year of AI Agents”, autonomous systems represent the practical application of these principles. AI agents that perceive their environment and take actions independently are now moving from experimental technology to mainstream business tools. This evolution builds on reinforcement learning foundations—where systems learn through trial and error to determine optimal outcomes.
Artificial intelligence has evolved significantly over recent years. Early systems primarily made predictions based on labelled data, excelling at classification tasks but lacking the ability to take action or adapt to changing environments. Now, reinforcement learning has expanded from specialised applications like game playing into broader commercial use, creating what researchers call Action-Driven AI—systems that both analyse information and take appropriate actions based on that analysis.
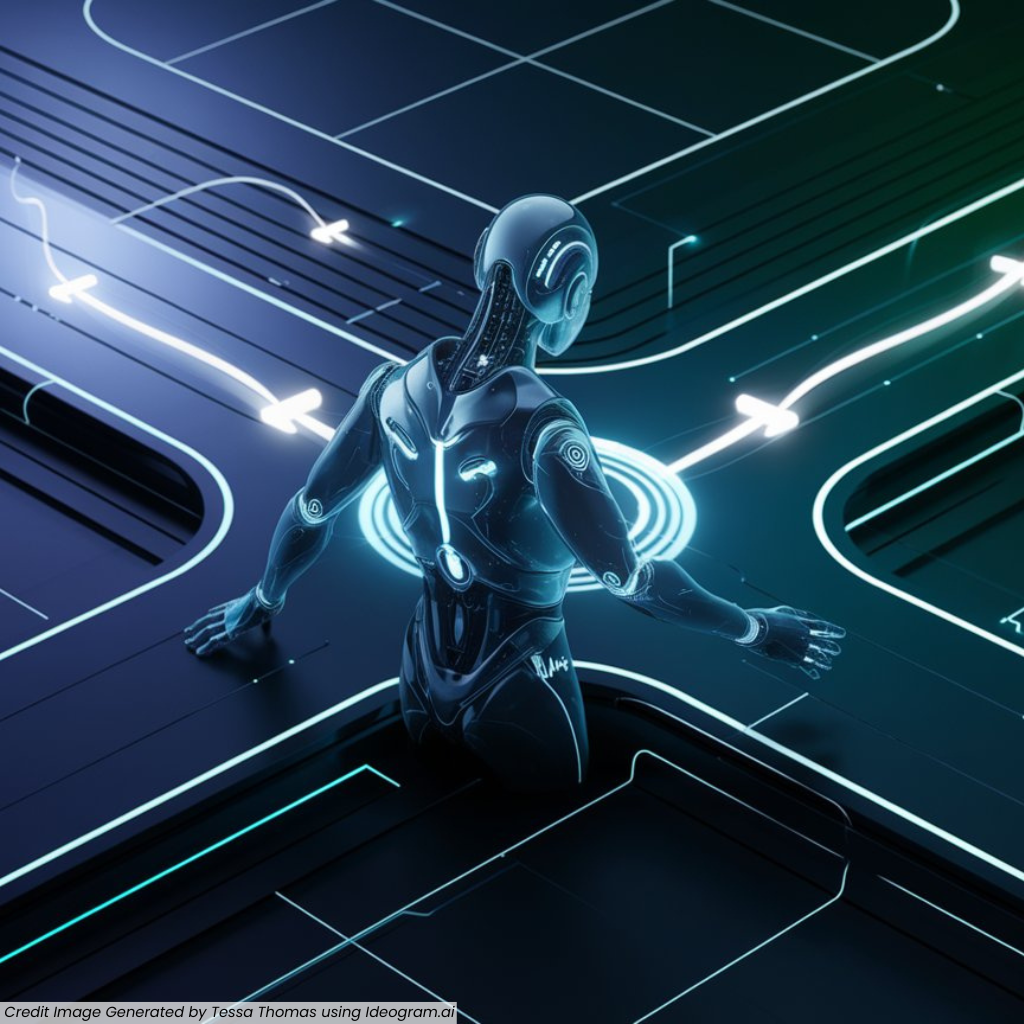
What is Reinforcement Learning & Why Should You Care?
Reinforcement learning (RL) represents a different approach to artificial intelligence compared to traditional methods. While supervised learning relies on historical data to make predictions, reinforcement learning creates systems that interact with their environment, learn from outcomes, and adapt their strategies over time.
Compared to rule-based automation, which operates using pre-programmed instructions, reinforcement learning enables adaptive decision-making. This capability allows AI systems to navigate complex situations where static, predefined rules might be insufficient.
This technology functions as a self-improving agent that learns through trial and error, figuring out which actions lead to the best outcomes without explicit programming.
Reinforcement learning applications are now widespread, from optimised ad delivery systems to intelligent trading algorithms. Tasks that once required constant human oversight now happen autonomously with minimal supervision.
For small and medium-sized businesses, this means you can now:
- Personalise customer experiences without manual intervention
- Optimise complex business processes automatically
- Create adaptive systems that improve over time
- Solve problems too complex for traditional programming approaches
The Key Components: How Reinforcement Learning Works
Reinforcement learning has become an important approach for many advanced AI applications. The technology consists of several key components that work together to create effective learning systems:
Policy:
- Maps the environment to specific actions at specific points
- Acts like a decision-making rulebook for the AI agent
- Evolves over time as the agent learns what works best
Reward Signal:
- Provides the goal, such as increasing sales or reducing costs
- Different values can be assigned to different outcomes
- Guides the agent toward desirable behaviours
Exploration-Exploitation:
- Systems must balance discovering new knowledge versus using what they already know
- Advanced algorithms automatically adjust this balance based on confidence levels
- Modern systems use sophisticated techniques to optimise this trade-off
The Major Applications: Where Reinforcement Learning Shines
Reinforcement learning has found applications across various industries. In transportation, self-driving vehicles use these techniques for autonomous navigation through complex environments, constantly improving through both simulation and real-world feedback.
Financial institutions apply reinforcement learning for trading algorithms and fraud detection systems that adapt to changing market conditions. Marketing teams leverage the technology for personalised customer experiences, including ad delivery and dynamic pricing models that respond to consumer behavior.
Energy companies are implementing reinforcement learning for smart grid optimisation to reduce costs and emissions, while also improving building management systems that learn usage patterns to maximise efficiency. Each industry finds ways to harness the adaptive nature of these systems to address their unique challenges.
Challenges & Limitations of Reinforcement Learning
While reinforcement learning is transforming industries, it comes with certain challenges. The technology often requires significant data and computational resources, making implementation costly for some organisations. There are also inherent risks in the exploration phase, as systems must try different actions that can lead to mistakes—particularly concerning in high-stakes environments.
Ethical considerations arise when AI systems make autonomous decisions, including questions about fairness in financial markets or potential bias in automated processes. Many reinforcement learning breakthroughs happen in simulations, but transitioning to real-world applications requires extensive fine-tuning and safety measures.
For businesses considering this technology, hybrid approaches that combine reinforcement learning with traditional machine learning methods can help mitigate risks while maximising benefits.
The Future: What's Next for Reinforcement Learning?
Reinforcement learning continues to evolve through breakthroughs in offline learning and integration with other AI technologies. The transition from academic research to commercial applications has been notable, with these systems providing measurable business value across multiple industries.
One promising direction is combining reinforcement learning with large language models (advanced AI systems that can understand and generate text), creating systems that understand context and take optimal actions. Meanwhile, increased accessibility through cloud platforms is allowing organisations of all sizes to implement these solutions without requiring specialised expertise or substantial computational resources.